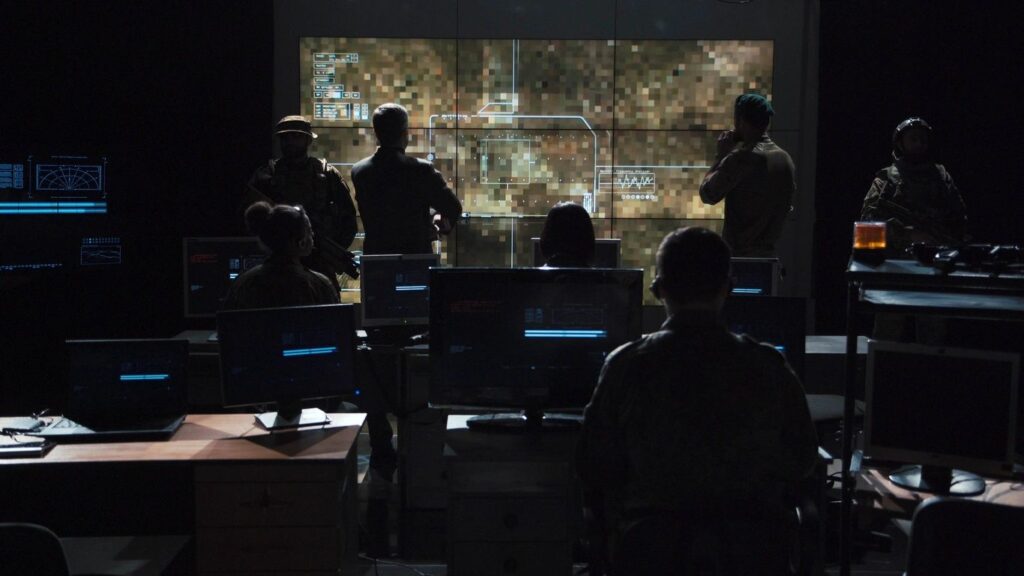
Artificial intelligence (AI) has the potential to transform our world and solve many of our most pressing problems. From healthcare to transportation, from education to the environment, AI is being used to improve outcomes, increase efficiency, and drive innovation. However, as with any technology, AI also has a dark side, and one of its biggest challenges is addressing bias and discrimination in machine learning. In this blog post, we’ll explore the issue of bias in AI and discuss strategies for addressing this critical challenge.
What is Bias in AI?
Bias in AI refers to the phenomenon where machine learning algorithms produce results that are systematically inaccurate or unfair. Bias can arise from a variety of sources, including biased data sets, flawed algorithms, and human bias in decision-making. When left unchecked, bias in AI can lead to discrimination, unfair treatment, and perpetuate existing social and economic inequalities.
Examples of Bias in AI
One well-known example of bias in AI is the case of facial recognition technology. Studies have shown that facial recognition algorithms are often less accurate for people with darker skin tones, leading to false positives and false negatives. This is because the data sets used to train these algorithms are often skewed towards lighter-skinned individuals, leading to biased outcomes.
Another example is the use of predictive policing algorithms, which have been criticized for perpetuating racial profiling and discrimination. These algorithms use historical crime data to predict where crimes are likely to occur in the future, but this data is often biased towards certain neighborhoods and demographics, leading to unfair and inaccurate predictions.
Addressing Bias in AI
Addressing bias in AI requires a multifaceted approach that involves both technical and ethical considerations. Here are some strategies for addressing this critical challenge:
- Diversify Data Sets: To reduce bias in AI, it’s important to use diverse data sets that accurately represent different populations and perspectives. This can help ensure that algorithms are trained on a broad range of examples, reducing the risk of bias.
- Evaluate Algorithms: It’s important to regularly evaluate machine learning algorithms to ensure that they are producing accurate and fair results. This involves testing algorithms on diverse data sets and examining the outcomes to identify any potential biases.
- Foster Ethical Decision-Making: It’s important to incorporate ethical considerations into the design and development of AI systems. This involves considering the potential impact of AI on different populations and ensuring that systems are designed with fairness and equity in mind.
- Increase Diversity in AI: Increasing diversity in the AI industry can help reduce bias by bringing new perspectives and experiences to the table. This involves promoting diversity and inclusion in hiring and creating programs to encourage underrepresented groups to enter the field.
In conclusion, bias and discrimination in AI are significant challenges that must be addressed if we are to realize the full potential of this technology. By diversifying data sets, evaluating algorithms, fostering ethical decision-making, and increasing diversity in the AI industry, we can help mitigate the impact of bias and build more equitable and inclusive AI systems. By doing so, we can ensure that AI serves as a force for good and helps us build a better world for all.